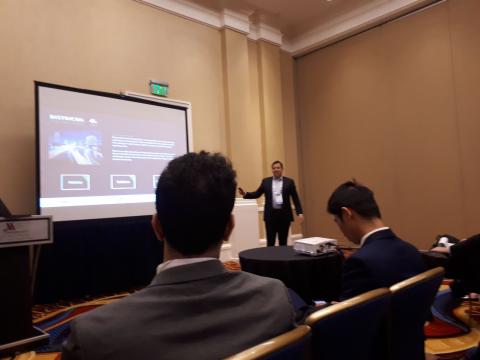
Districon joined a presentation of AIMMS during the INFORMS Conference 2018 in Baltimore.
Our colleague Sander van Lokven joined a presentation of Gertjan de Lange (SVP Connecting Business & Optimization @ AIMMS) at the INFORMS – 2018 Business Analytics Conference in Baltimore. Sander presented 2 project case examples to support Gertjan de Lange’s presentation on:
The Intersection of OR and Data Science – Opportunities, Challenges, and Innovation
In the history of almost 30 years in the market, AIMMS has executed some pivotal changes that were needed to ensure the value of optimization could be fully realized by their customers. Driven by market requirements, customer feedback and innovation initiatives, AIMMS remain laser focused on continuing to bring more value each day and broadening awareness of the benefits of optimization. Looking at the development of the Data Science industry, they realize there is a big opportunity for all of them and their customers. However, it also creates challenges as the modeling paradigms might conflict and the conversations shift. As things evolve there are likely to be significant implications and questions that may arise as new developments in data science, the consumerization of technology and software as a service, force AIMMS to think differently on how they service their community of partners and customers.
Districon presented two projects where in practice data science and OR are combined to improve the quality of the decision. The quality of optimization models output is dependent on the quality of the data you provide (garbage in is garbage out). Data science is used in practice to improve the quality of the data. Presented example from fashion industry illustrated the use of forecasting on product group level, and continuous data improvement by adjusting the forecast on product/item level. Next step in this process is to involve machine learning to take certain patterns into account which are not spotted by the current used statistical models.
The second example (Profit based business planning and scheduling @ Nampak) illustrated the use of data science in a decision process where the optimization output is reviewed (in this case multiple scenarios, e.g. Maximum profit scenario versus Maximum customer satisfaction with lowest missed order volume). This review can automated or manual. In the illustrated example the review is manually and may lead to adjusted constraints or objective (e.g. Maximize the customer satisfaction, given that the profit must be within 10% of the Maximum profit output).